What is: Joint Alternative Hypothesis
What is: Joint Alternative Hypothesis
The Joint Alternative Hypothesis is a fundamental concept in the field of statistics, particularly in hypothesis testing. It represents a collective assertion about the parameters of multiple populations or groups being studied. Unlike the simple alternative hypothesis, which focuses on a single parameter, the joint alternative hypothesis encompasses a broader scope, allowing researchers to evaluate the relationships and differences among several variables simultaneously. This approach is particularly useful in complex data analysis scenarios where interactions between multiple factors are of interest.
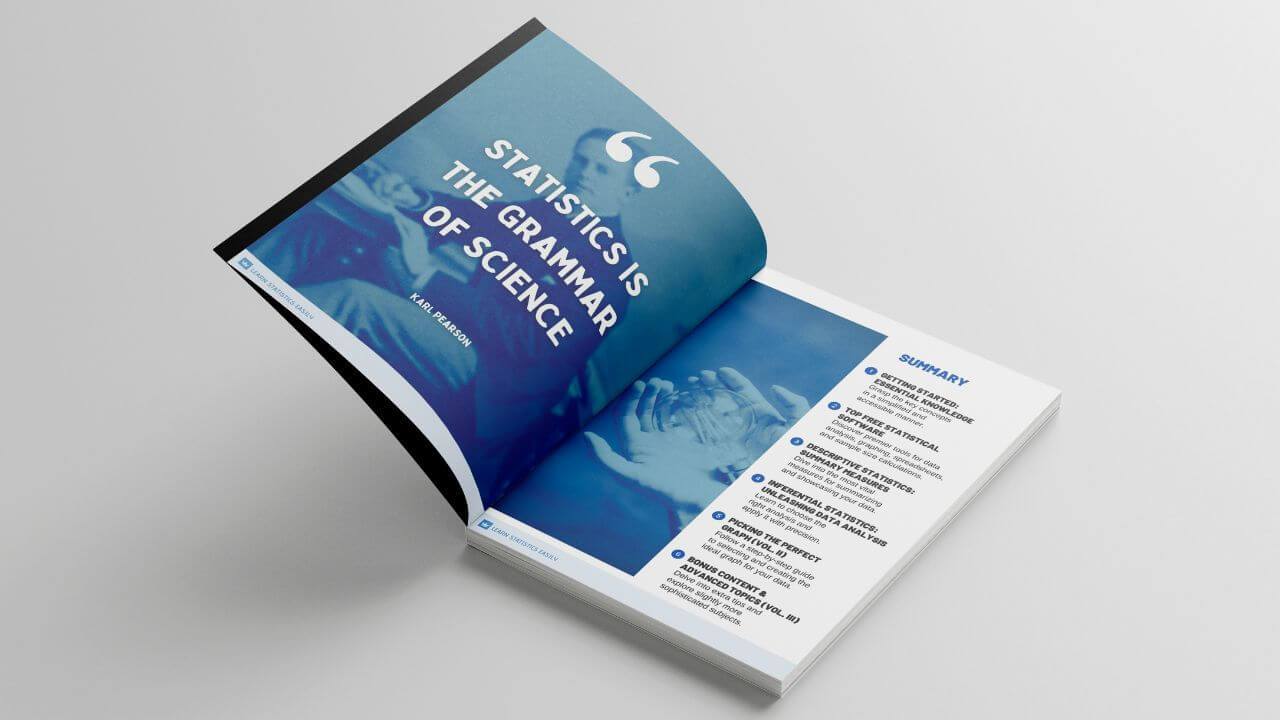
Transform Your Research with Data Analysis!
Enhance your research outcomes with data analysis. Learn the necessary techniques now!
In statistical terms, the Joint Alternative Hypothesis is often denoted as H1 or Ha, and it is contrasted with the null hypothesis (H0), which posits that there are no effects or differences among the groups being analyzed. The formulation of a joint alternative hypothesis can vary depending on the specific research question and the nature of the data. For instance, in a study examining the effects of different treatments on patient outcomes, the joint alternative hypothesis might state that at least one treatment leads to a different outcome compared to the others.
The testing of the Joint Alternative Hypothesis typically involves the use of statistical methods such as ANOVA (Analysis of Variance) or MANOVA (Multivariate Analysis of Variance). These techniques allow researchers to assess whether the observed data provide sufficient evidence to reject the null hypothesis in favor of the joint alternative hypothesis. The significance level, often set at 0.05, plays a crucial role in this decision-making process, determining the threshold for rejecting H0.
One of the key advantages of utilizing a Joint Alternative Hypothesis is its ability to capture the complexity of real-world phenomena. In many cases, variables do not operate in isolation; rather, they interact in ways that can significantly influence outcomes. By considering multiple hypotheses simultaneously, researchers can gain a more nuanced understanding of the data and the underlying processes at play. This holistic approach is particularly valuable in fields such as data science and epidemiology, where multifactorial influences are common.
Moreover, the formulation of a Joint Alternative Hypothesis necessitates careful consideration of the relationships among the variables involved. Researchers must define the specific parameters they wish to test and articulate the expected outcomes clearly. This clarity is essential for ensuring that the statistical tests employed are appropriate and that the results can be interpreted meaningfully. In this context, collaboration among statisticians, domain experts, and data analysts is often crucial for developing robust hypotheses.
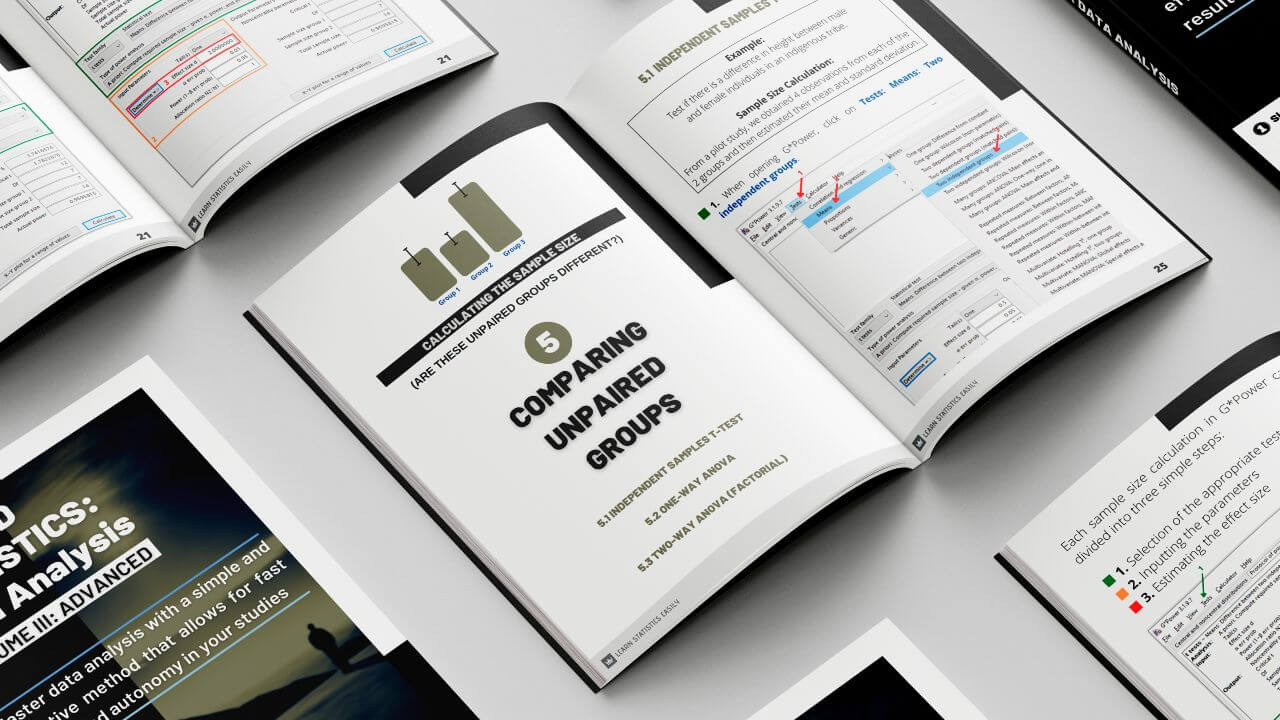
Learn Statistics for Data Analysis!
Demystify statistics and discover how to analyze your data efficiently.
In practice, the Joint Alternative Hypothesis can lead to more powerful statistical tests. By pooling information across multiple groups, researchers can increase the likelihood of detecting true effects when they exist. This is particularly important in studies with limited sample sizes, where the risk of Type II errors (failing to reject a false null hypothesis) is heightened. Consequently, the joint approach can enhance the overall validity and reliability of the research findings.
It is also important to note that the interpretation of results derived from testing a Joint Alternative Hypothesis can be complex. Researchers must be cautious in drawing conclusions, as the presence of significant results does not automatically imply that all tested parameters are different. Instead, post-hoc analyses may be necessary to identify which specific groups or conditions contributed to the observed effects. This additional layer of analysis is essential for providing actionable insights based on the research outcomes.
In summary, the Joint Alternative Hypothesis serves as a powerful tool in the arsenal of statisticians and data scientists. By allowing for the simultaneous testing of multiple parameters, it facilitates a deeper understanding of complex datasets and enhances the ability to draw meaningful conclusions. As the field of data analysis continues to evolve, the importance of robust hypothesis formulation and testing, including the use of joint alternatives, will remain a cornerstone of effective statistical practice.
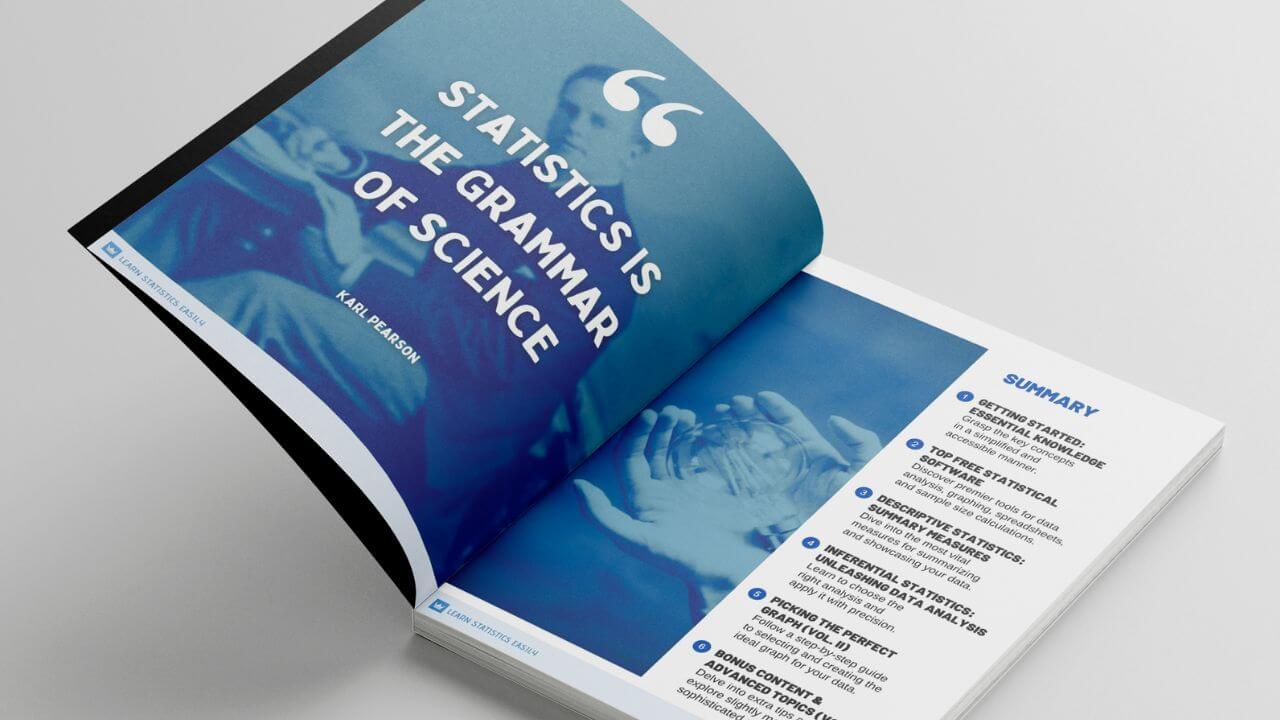
Analyze Data like a Professional!
Unravel the secrets of statistical analysis and enhance your scientific projects. Don’t miss this chance!