What is: K-Stat Analysis
What is K-Stat Analysis?
K-Stat Analysis, or K-statistic analysis, is a statistical method used to evaluate the properties of a dataset, particularly in the context of time series data. This technique focuses on the estimation of the K-statistics, which are a set of estimators that provide insights into the distribution and characteristics of the data. By applying K-Stat Analysis, researchers can identify patterns, trends, and anomalies that may not be immediately apparent through conventional statistical methods.
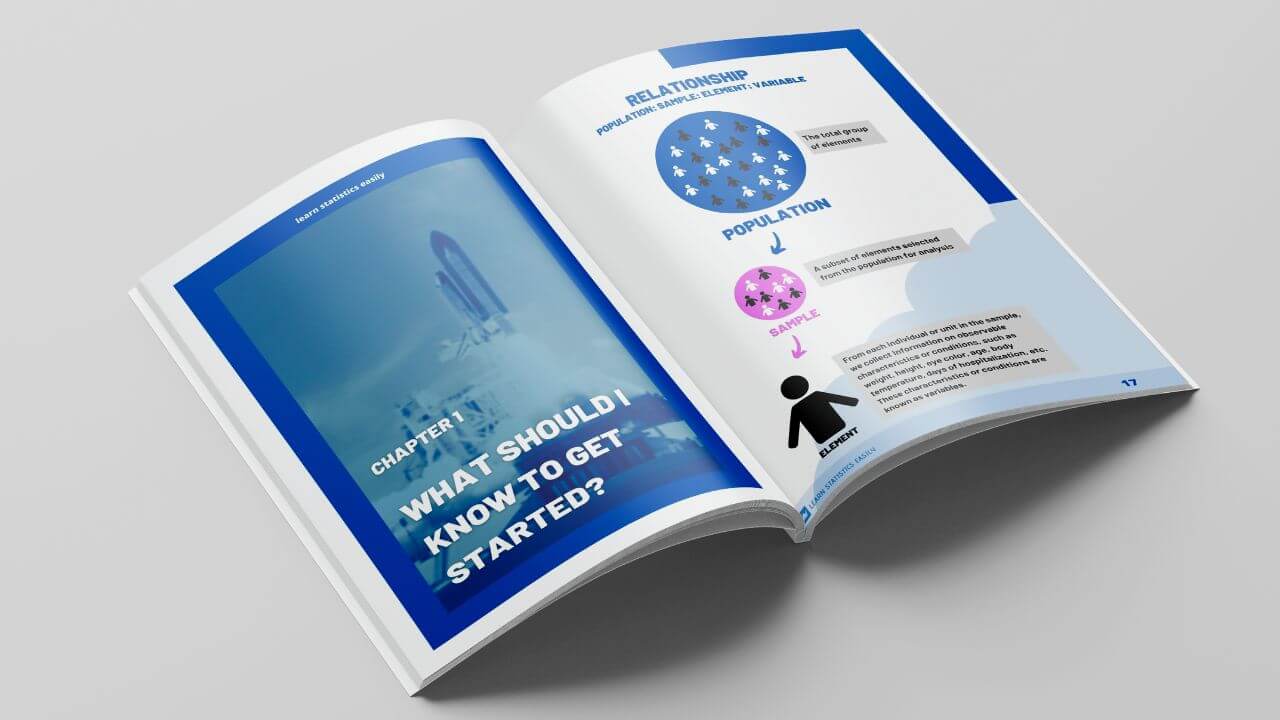
Learn Statistics for Data Analysis!
Demystify statistics and discover how to analyze your data efficiently.
Understanding K-Statistics
K-statistics are derived from sample moments and are particularly useful in the analysis of non-normal distributions. They are defined as a function of the sample moments, which are calculated from the data. The K-statistics provide a robust framework for assessing the skewness and kurtosis of a dataset, allowing analysts to understand the shape and behavior of the data distribution. This is crucial for making informed decisions based on statistical evidence.
Applications of K-Stat Analysis
K-Stat Analysis finds applications across various fields, including finance, economics, and environmental science. In finance, for example, K-statistics can help in assessing the risk and return profiles of investment portfolios. In environmental studies, K-Stat Analysis can be employed to analyze climate data, helping researchers understand trends in temperature changes or precipitation patterns over time. The versatility of K-Stat Analysis makes it a valuable tool in any data-driven decision-making process.
Advantages of K-Stat Analysis
One of the primary advantages of K-Stat Analysis is its ability to handle non-normal data distributions effectively. Traditional statistical methods often assume normality, which can lead to inaccurate conclusions when applied to real-world data. K-Stat Analysis, on the other hand, provides a more flexible approach, allowing analysts to derive meaningful insights from datasets that exhibit skewness or heavy tails. This robustness enhances the reliability of the analysis and the decisions based on it.
How to Perform K-Stat Analysis
Performing K-Stat Analysis involves several steps, starting with data collection and preprocessing. Once the data is prepared, analysts calculate the sample moments, which are then used to derive the K-statistics. Various software tools and programming languages, such as R and Python, offer libraries and functions specifically designed for K-Stat Analysis, making it accessible for practitioners. Visualization techniques can also be employed to illustrate the findings, aiding in the interpretation of the results.
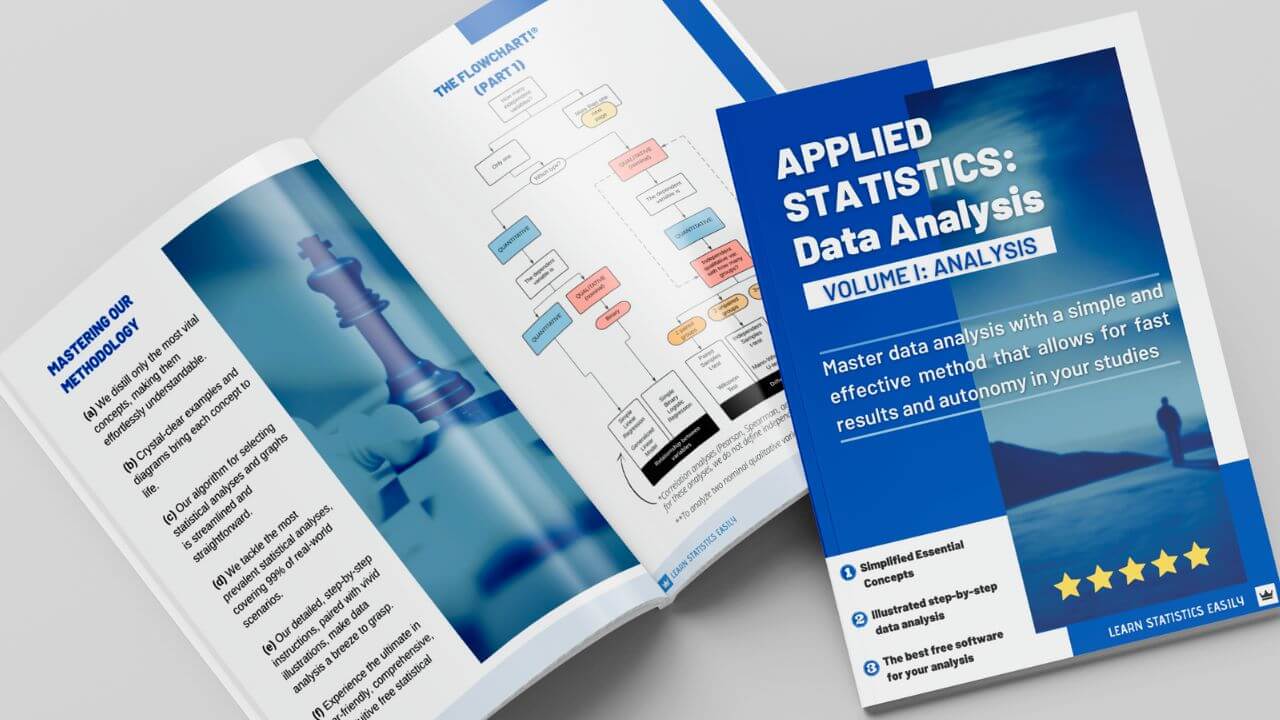
Be a Master of Data Analysis!
Master data analysis techniques and stand out in your research field. Learn in a simple way!
Interpreting K-Stat Results
Interpreting the results of K-Stat Analysis requires a solid understanding of the underlying statistical principles. Analysts must consider the values of the K-statistics in relation to the expected distribution characteristics. For instance, a positive skewness indicates that the tail on the right side of the distribution is longer or fatter than the left side, while a negative skewness suggests the opposite. Similarly, kurtosis values can indicate whether the data has heavier or lighter tails compared to a normal distribution.
Limitations of K-Stat Analysis
Despite its advantages, K-Stat Analysis is not without limitations. One significant challenge is the sensitivity of K-statistics to outliers, which can skew the results and lead to misleading interpretations. Additionally, while K-Stat Analysis is powerful for certain types of data, it may not be the best choice for all datasets. Analysts must carefully assess the appropriateness of K-Stat Analysis based on the specific characteristics of the data they are working with.
Comparing K-Stat Analysis with Other Methods
K-Stat Analysis is often compared to other statistical methods, such as traditional hypothesis testing and regression analysis. While these methods have their strengths, K-Stat Analysis offers unique advantages when dealing with non-normal data distributions. It provides a more nuanced understanding of the data’s behavior, particularly in cases where conventional methods may fall short. This comparative advantage makes K-Stat Analysis an essential tool in the statistician’s toolkit.
Future Trends in K-Stat Analysis
As data science continues to evolve, the methodologies surrounding K-Stat Analysis are also expected to advance. The integration of machine learning techniques with K-Stat Analysis could enhance its predictive capabilities, allowing for more sophisticated analyses of complex datasets. Furthermore, as the availability of big data increases, the need for robust statistical methods like K-Stat Analysis will become even more critical in extracting actionable insights from vast amounts of information.
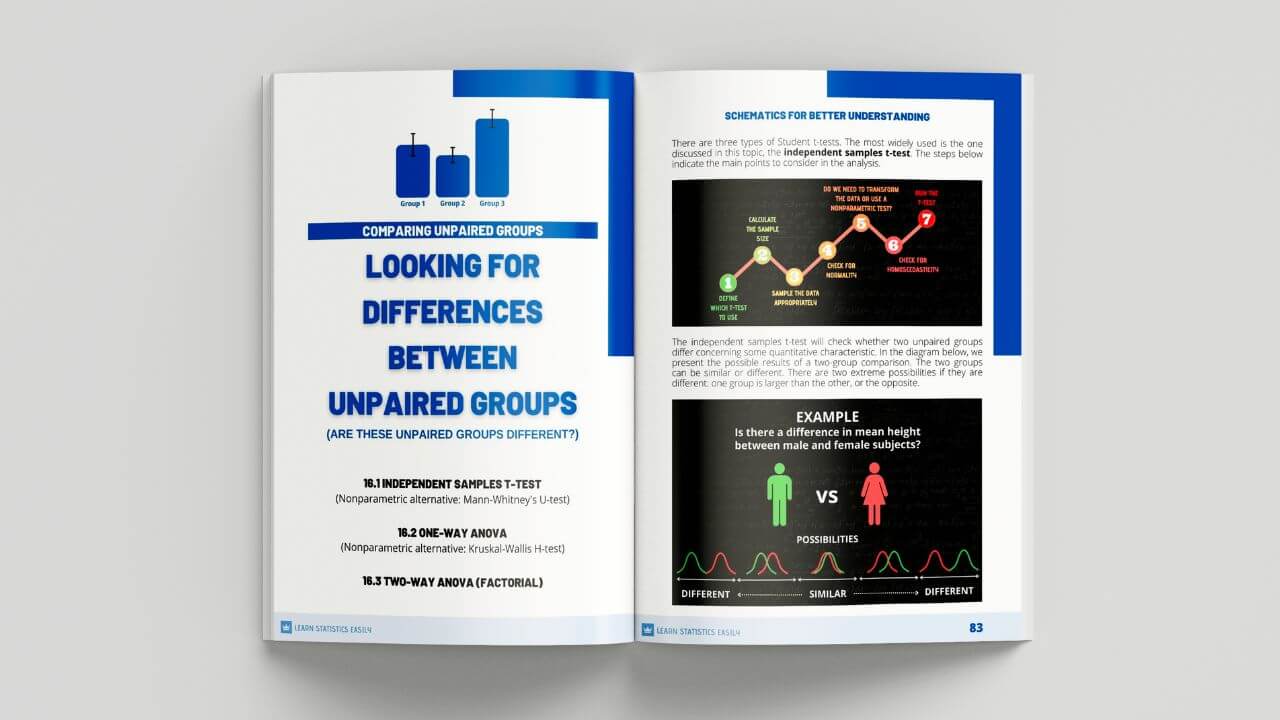
Make a Difference with Data Analysis!
Find out how data analysis can boost your scientific work. Take this unique opportunity!