What is: K-Statistical Inference
What is K-Statistical Inference?
K-Statistical Inference is a specialized branch of statistics that focuses on making predictions or generalizations about a population based on a sample. This method is particularly useful when dealing with large datasets where it is impractical to collect data from every individual in the population. By employing K-Statistical Inference, researchers can derive insights and make informed decisions while minimizing the risk of error.
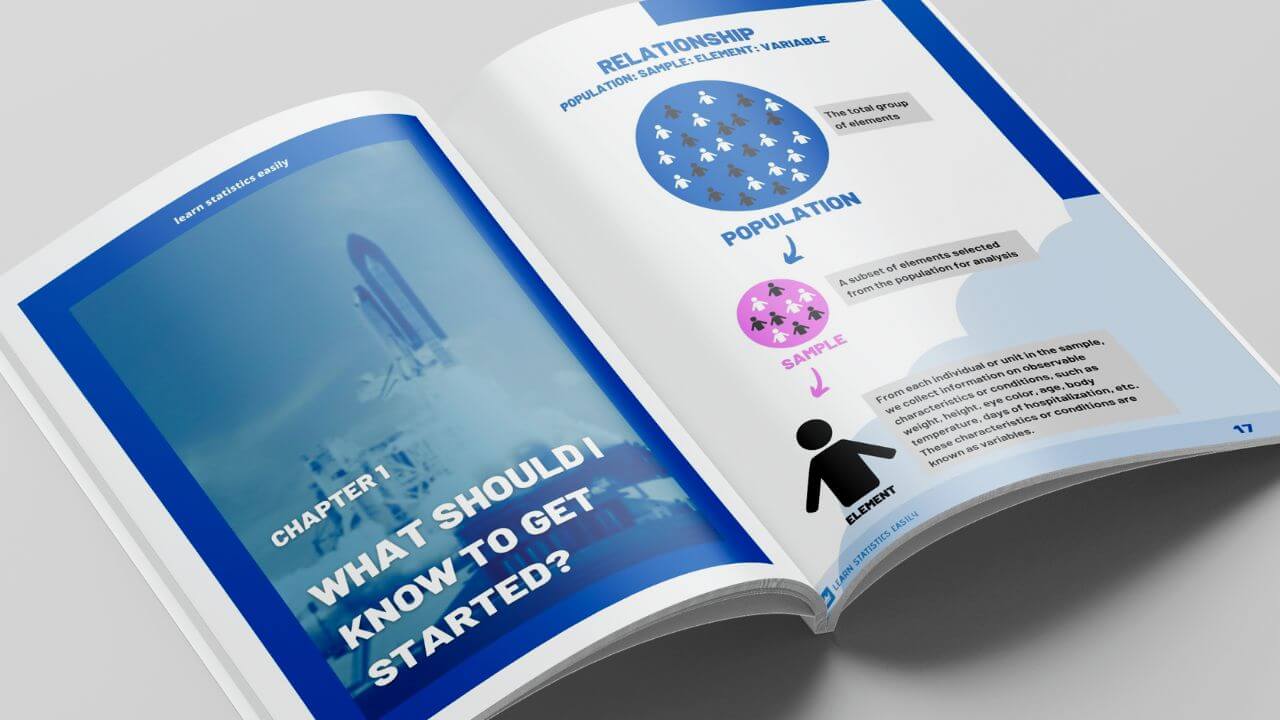
Learn Statistics for Data Analysis!
Demystify statistics and discover how to analyze your data efficiently.
Understanding the Basics of K-Statistical Inference
The foundation of K-Statistical Inference lies in the principles of probability and sampling. It involves using statistical models to estimate population parameters, such as means or proportions, based on sample statistics. This process often includes the application of various statistical tests to determine the reliability of the inferences made. The ‘K’ in K-Statistical Inference typically refers to the number of categories or groups being analyzed, which can significantly impact the results and interpretations.
Key Components of K-Statistical Inference
Several key components are essential to K-Statistical Inference, including hypothesis testing, confidence intervals, and p-values. Hypothesis testing allows researchers to assess the validity of their assumptions about a population. Confidence intervals provide a range of values within which the true population parameter is likely to fall, while p-values help determine the statistical significance of the results. Together, these components form the backbone of K-Statistical Inference, enabling robust analysis and interpretation.
Applications of K-Statistical Inference
K-Statistical Inference is widely applied across various fields, including social sciences, healthcare, and market research. For instance, in clinical trials, researchers use K-Statistical Inference to evaluate the effectiveness of new treatments by comparing sample groups. Similarly, businesses leverage this statistical approach to analyze consumer behavior and preferences, guiding marketing strategies and product development. The versatility of K-Statistical Inference makes it a valuable tool in data-driven decision-making.
Challenges in K-Statistical Inference
Despite its advantages, K-Statistical Inference is not without challenges. One significant issue is the potential for sampling bias, which can lead to inaccurate conclusions if the sample is not representative of the population. Additionally, the assumptions underlying statistical models must be carefully considered, as violations can compromise the validity of the inferences drawn. Researchers must remain vigilant in addressing these challenges to ensure the integrity of their findings.
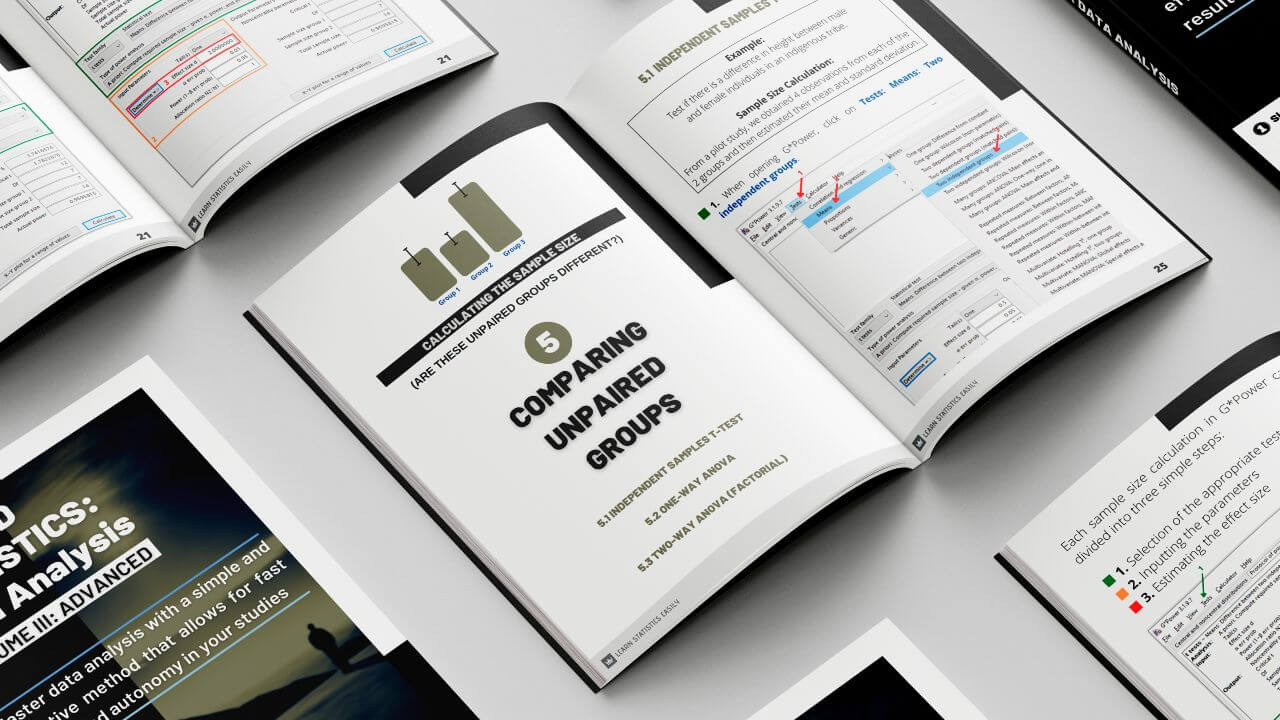
Become a Data Analysis Expert!
Expand your knowledge in data analysis and stand out in your academic field. Start your journey right now!
Advanced Techniques in K-Statistical Inference
As the field of statistics evolves, so do the techniques used in K-Statistical Inference. Advanced methods, such as Bayesian inference and machine learning algorithms, are increasingly being integrated into traditional statistical frameworks. These approaches allow for more nuanced analyses and can accommodate complex datasets that may not fit conventional models. By embracing these advancements, researchers can enhance the accuracy and applicability of their inferences.
Importance of Sample Size in K-Statistical Inference
The size of the sample plays a crucial role in K-Statistical Inference. A larger sample size generally leads to more reliable estimates of population parameters and reduces the margin of error. However, practical constraints often limit the size of samples that can be collected. Researchers must balance the need for sufficient data with the resources available, making strategic decisions about sample selection and size to optimize their findings.
Interpreting Results from K-Statistical Inference
Interpreting the results of K-Statistical Inference requires a solid understanding of statistical concepts and the context of the data. Researchers must communicate their findings clearly, highlighting the implications of their inferences while acknowledging any limitations. Effective interpretation also involves considering the practical significance of the results, not just the statistical significance, to ensure that the insights gained are actionable and relevant.
The Future of K-Statistical Inference
Looking ahead, K-Statistical Inference is poised to play an increasingly important role in data analysis and decision-making. As the volume of data continues to grow, the need for robust statistical methods will become even more critical. Innovations in technology and computational power will further enhance the capabilities of K-Statistical Inference, enabling researchers to tackle more complex problems and derive deeper insights from their data.
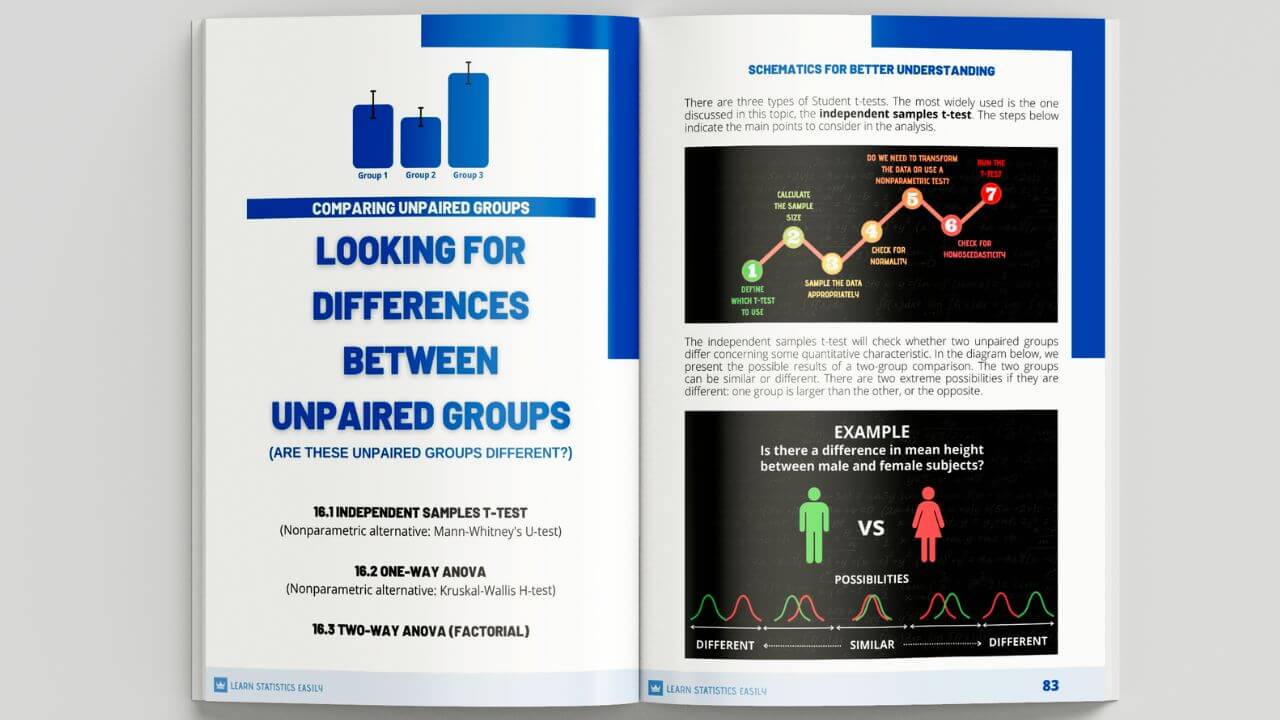
Make a Difference with Data Analysis!
Find out how data analysis can boost your scientific work. Take this unique opportunity!