What is: K-T-Test
What is K-T-Test?
The K-T-Test, or Kruskal-Wallis Test, is a non-parametric statistical method used to determine if there are statistically significant differences between the medians of three or more independent groups. This test is particularly useful when the assumptions of normality and homogeneity of variance are not met, making it a robust alternative to the one-way ANOVA. The K-T-Test is widely applied in various fields, including psychology, medicine, and social sciences, where researchers often deal with ordinal data or non-normally distributed interval data.
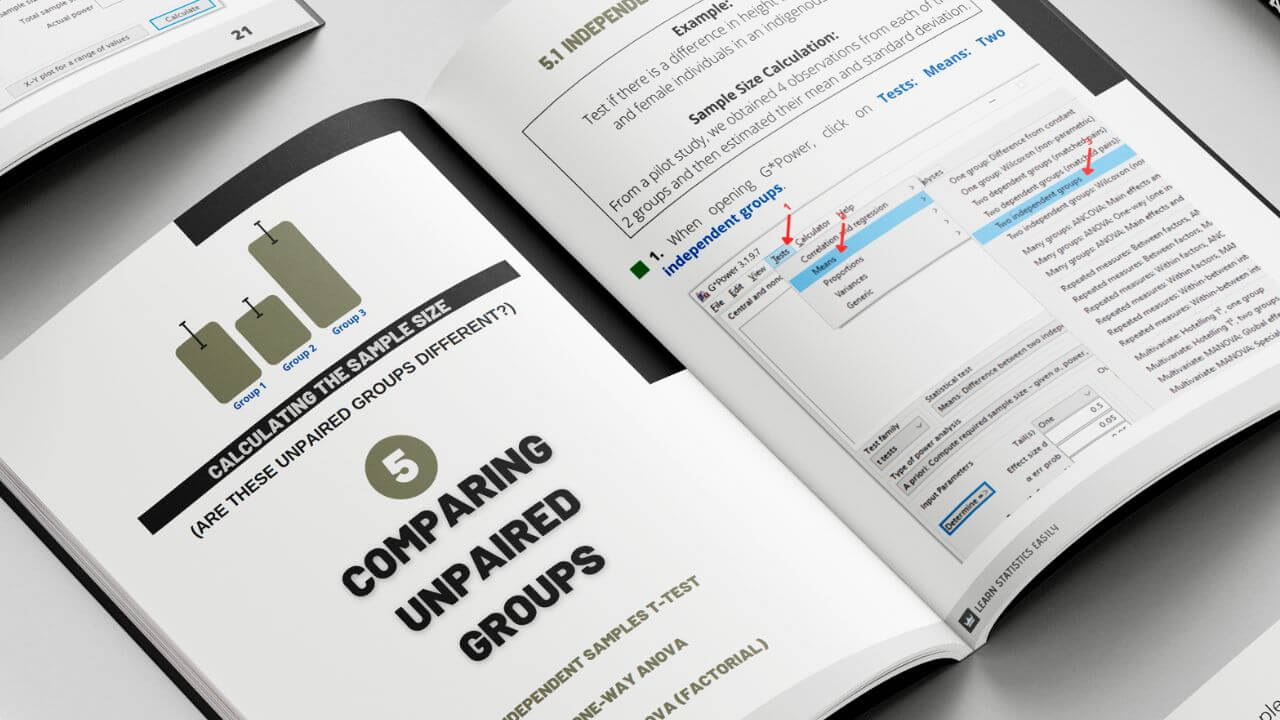
Simplify the Analysis of Your Data!
Gain mastery of statistics and analyze your data with confidence. Start your journey right now!
Understanding the K-T-Test
The K-T-Test operates on the principle of ranking the data from all groups together, rather than analyzing the raw data. Each observation is assigned a rank, and the test statistic is calculated based on these ranks. The null hypothesis of the K-T-Test posits that all groups have the same median, while the alternative hypothesis suggests that at least one group differs significantly from the others. This method allows researchers to identify differences in distributions without assuming a specific distribution shape.
When to Use the K-T-Test
The K-T-Test is particularly advantageous when dealing with small sample sizes or when the data does not meet the assumptions required for parametric tests. Researchers should consider using the K-T-Test when they have three or more independent groups and when the data is ordinal or continuous but not normally distributed. It is also useful in experimental designs where treatments are applied to different groups, and the outcome measures are not normally distributed.
Assumptions of the K-T-Test
While the K-T-Test is a non-parametric test, it still has some assumptions that must be met for the results to be valid. Firstly, the observations must be independent of each other, meaning that the data collected from one group should not influence the data from another group. Secondly, the dependent variable should be measured on at least an ordinal scale. Lastly, the groups being compared should have similar shapes of distribution, although this assumption is less stringent than that of parametric tests.
Calculating the K-T-Test
The calculation of the K-T-Test involves several steps. First, all data points from the different groups are combined and ranked. Next, the sum of ranks for each group is calculated. The K-T-Test statistic is then computed using these rank sums, which is compared against a chi-squared distribution to determine the significance of the results. The degrees of freedom for the test are calculated as the number of groups minus one.
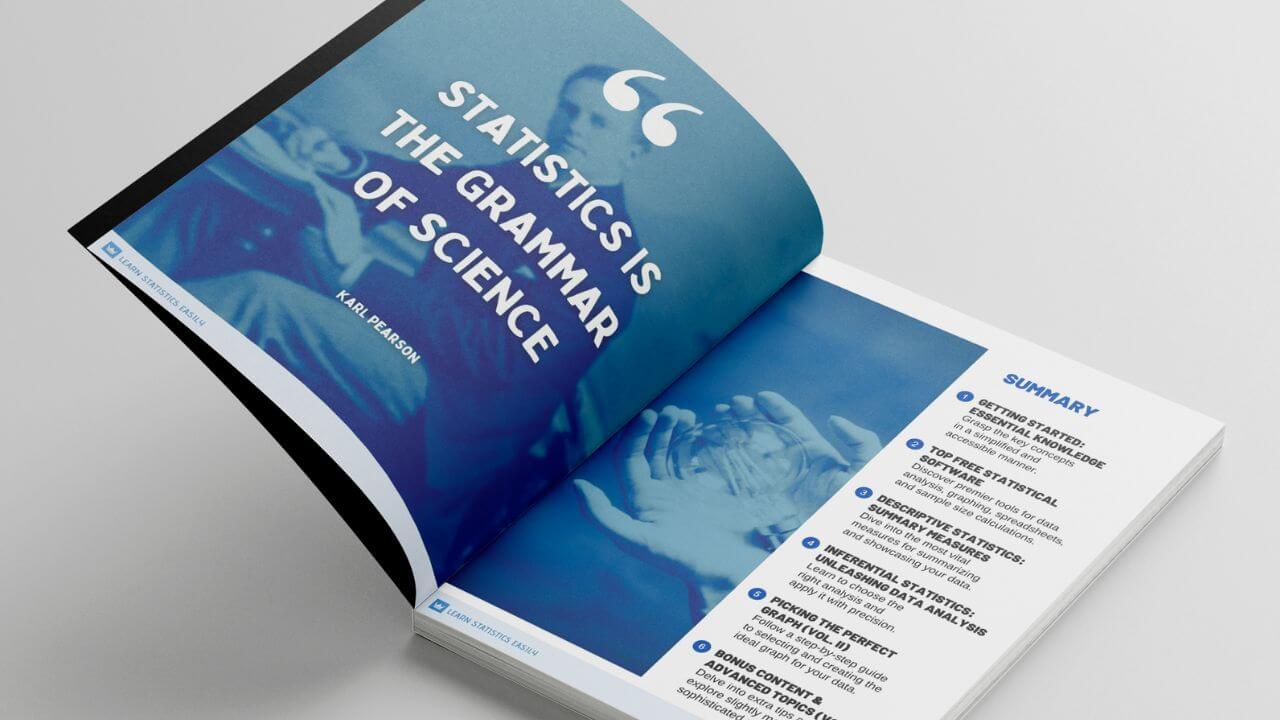
Simplify the Analysis of Your Data!
Gain mastery of statistics and analyze your data with confidence. Start your journey right now!
Interpreting K-T-Test Results
When interpreting the results of the K-T-Test, researchers look at the p-value associated with the test statistic. A p-value less than the chosen significance level (commonly 0.05) indicates that there is a statistically significant difference between the groups. If the null hypothesis is rejected, post-hoc tests may be conducted to identify which specific groups differ from each other. Common post-hoc tests include Dunn’s test and the Conover test, which help to pinpoint the exact nature of the differences.
Applications of the K-T-Test
The K-T-Test is utilized in various research scenarios. For instance, in clinical trials, it can be used to compare the effectiveness of different treatments across multiple patient groups. In educational research, it may be applied to assess the performance of students from different teaching methods. Additionally, in market research, the K-T-Test can help analyze consumer preferences across different demographic groups, providing valuable insights for businesses.
Limitations of the K-T-Test
Despite its advantages, the K-T-Test has limitations. One major drawback is that it only indicates whether there is a difference among groups but does not specify where those differences lie. Additionally, the K-T-Test is less powerful than parametric tests when the assumptions of those tests are met. This means that in cases where the data is normally distributed, using a one-way ANOVA may yield more reliable results.
Conclusion on K-T-Test
In summary, the K-T-Test is a valuable statistical tool for researchers dealing with non-parametric data. Its ability to handle ordinal data and its robustness against violations of normality make it an essential method in the toolkit of data analysts and scientists. Understanding when and how to apply the K-T-Test can lead to more accurate and meaningful insights in various fields of research.
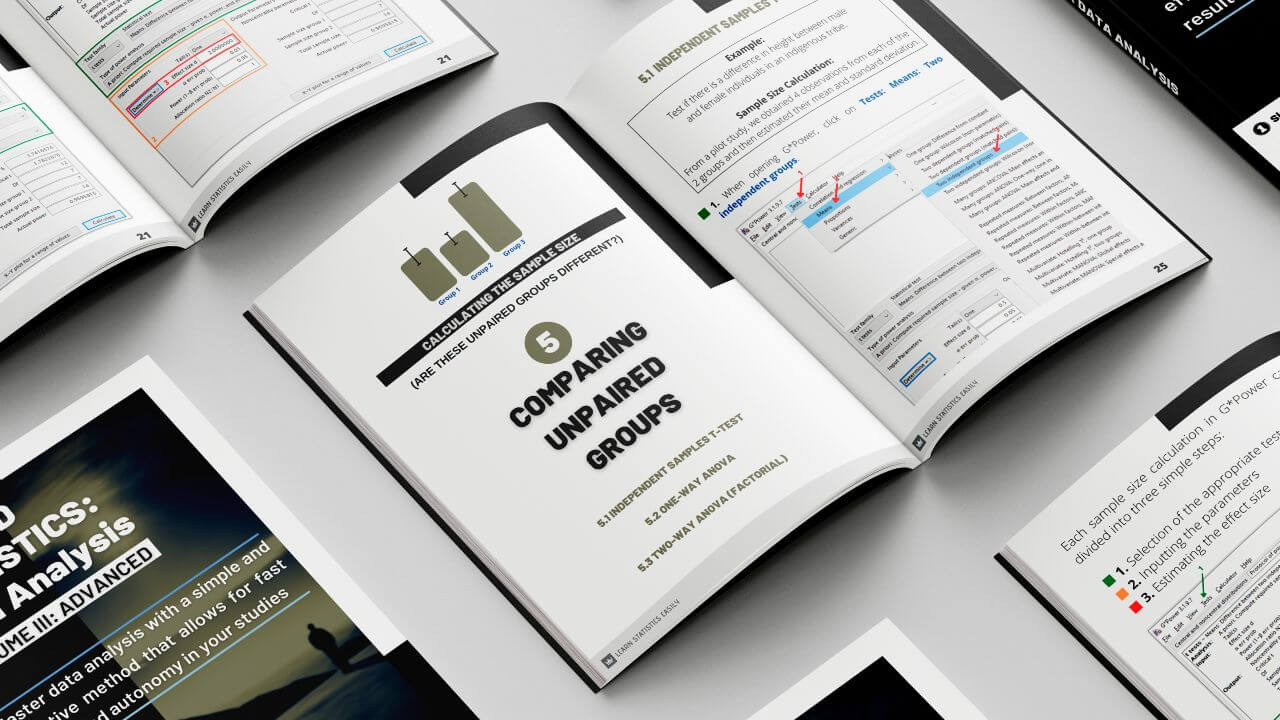
Simplify the Analysis of Your Data!
Gain mastery of statistics and analyze your data with confidence. Start your journey right now!