What is: Power Curve
What is a Power Curve?
The power curve is a graphical representation that illustrates the relationship between the power output of a system and the variables affecting it, such as wind speed in wind turbines or the performance of statistical models. This curve is essential in various fields, including statistics, data analysis, and data science, as it helps in understanding the efficiency and effectiveness of different processes. By analyzing the power curve, researchers and analysts can identify optimal operating conditions and make informed decisions based on empirical data.
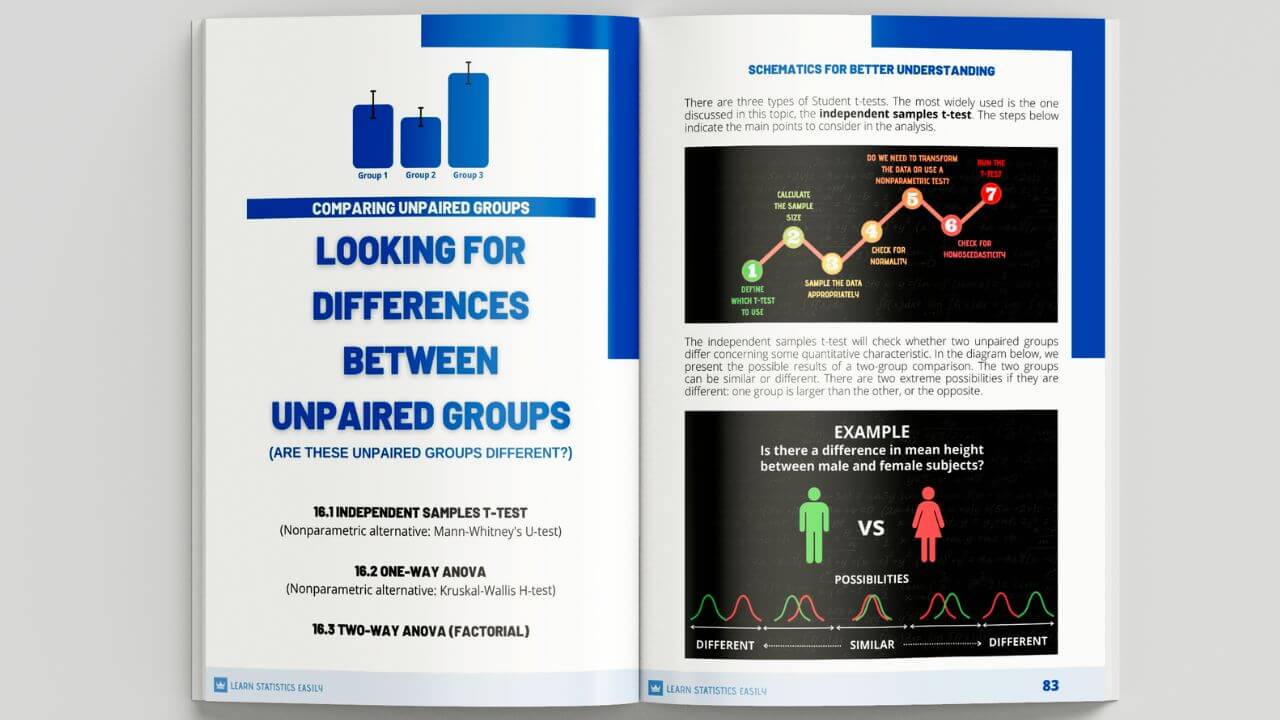
Be a Master of Data Analysis!
Master data analysis techniques and stand out in your research field. Learn in a simple way!
Components of the Power Curve
A power curve typically consists of several key components, including the x-axis, which represents the independent variable (e.g., wind speed), and the y-axis, which indicates the dependent variable (e.g., power output). The curve itself is usually nonlinear, demonstrating how power output changes with varying levels of the independent variable. Understanding these components is crucial for interpreting the power curve accurately and applying it to real-world scenarios.
Applications of Power Curves in Data Science
In data science, power curves are utilized to evaluate the performance of predictive models. For instance, they can help in assessing the trade-offs between true positive rates and false positive rates in classification tasks. By plotting the power curve, data scientists can visualize the effectiveness of different models and select the one that offers the best balance between sensitivity and specificity. This application is particularly important in fields such as healthcare, finance, and marketing, where decision-making relies heavily on accurate predictions.
Interpreting the Power Curve
Interpreting a power curve involves understanding the shape and characteristics of the curve itself. A steep curve indicates a high sensitivity to changes in the independent variable, while a flatter curve suggests a more stable relationship. Analysts must also consider the area under the curve (AUC), which provides a quantitative measure of the model’s performance. A higher AUC value signifies better predictive accuracy, making it a critical factor in model evaluation.
Power Curves in Wind Energy
In the context of wind energy, the power curve of a wind turbine is crucial for determining its efficiency and energy output at various wind speeds. The curve typically shows that power output increases with wind speed until it reaches a maximum point, after which it levels off or decreases. This information is vital for energy producers to optimize turbine placement and predict energy generation, ultimately contributing to more sustainable energy practices.
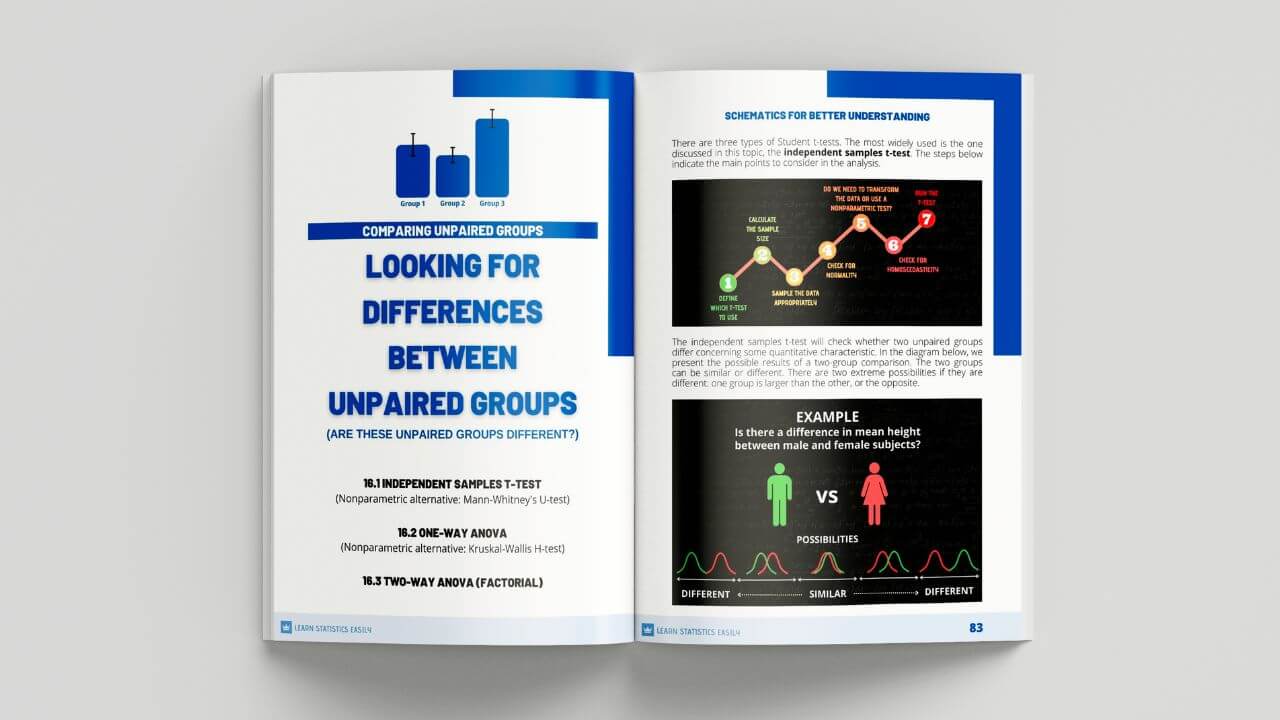
Simplify Data Analysis!
Don’t let statistics intimidate you. Learn to analyze your data with ease and confidence. Read more!
Factors Influencing Power Curves
Several factors can influence the shape and characteristics of a power curve, including environmental conditions, system design, and operational parameters. In statistical modeling, the choice of algorithms, feature selection, and data quality can significantly impact the power curve’s representation. Understanding these factors allows analysts to refine their models and improve overall performance, leading to more accurate predictions and insights.
Power Curves and Statistical Analysis
In statistical analysis, power curves are often used in hypothesis testing to determine the likelihood of correctly rejecting a null hypothesis. The power of a test is defined as the probability of correctly identifying an effect when it exists, and the power curve illustrates how this probability changes with varying sample sizes or effect sizes. This application is essential for researchers to design experiments that are adequately powered to detect meaningful differences.
Limitations of Power Curves
While power curves are valuable tools, they also have limitations. For instance, they may not account for all variables influencing the system, leading to oversimplified interpretations. Additionally, the accuracy of a power curve depends heavily on the quality of the data used to generate it. Analysts must be cautious in their interpretations and consider complementary analyses to validate their findings.
Future Trends in Power Curve Analysis
As technology advances, the analysis of power curves is expected to evolve significantly. The integration of machine learning and artificial intelligence into data analysis will enhance the ability to model complex relationships and improve predictive accuracy. Furthermore, real-time data collection and analysis will allow for more dynamic power curve assessments, enabling organizations to adapt quickly to changing conditions and optimize their operations effectively.
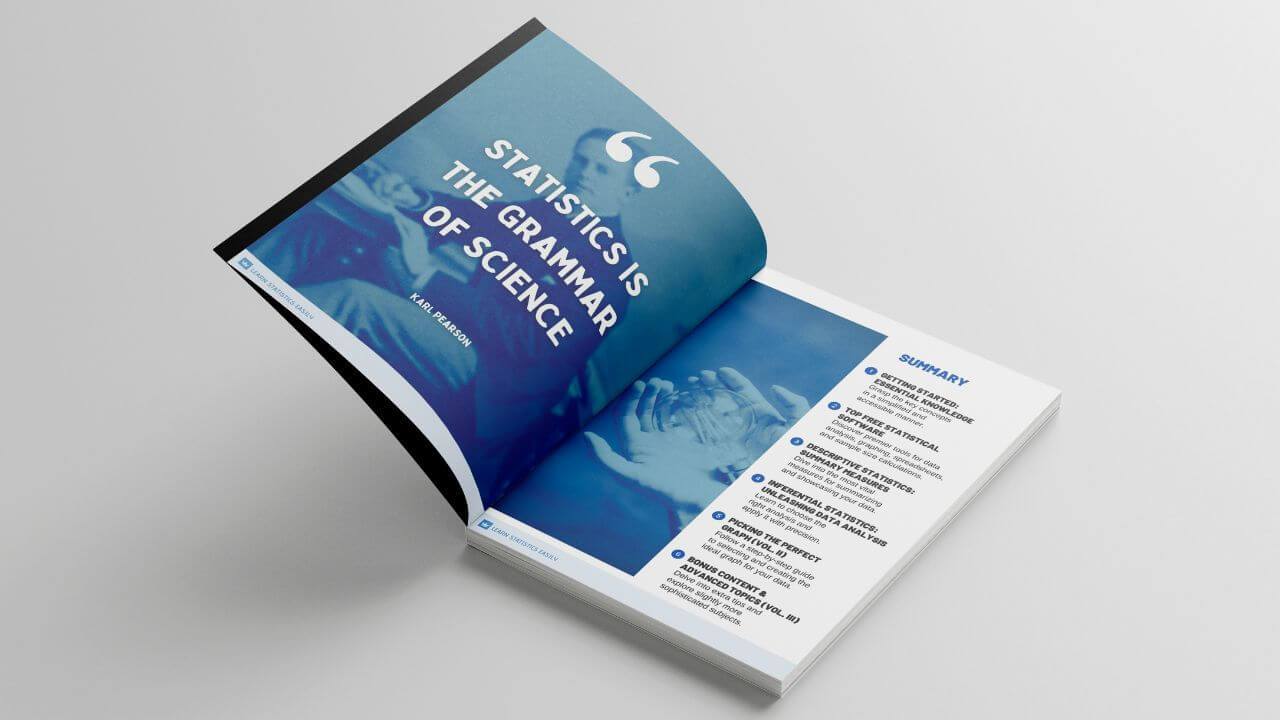
Simplify the Analysis of Your Data!
Gain mastery of statistics and analyze your data with confidence. Start your journey right now!