What is: Y-Outlier
What is Y-Outlier?
The term Y-Outlier refers to a specific type of outlier in statistical analysis, particularly in the context of regression models. In data science, outliers are data points that deviate significantly from the overall pattern of data. Y-Outliers are characterized by their unusual values in the dependent variable (Y) while the independent variables (X) remain within a normal range. Identifying Y-Outliers is crucial for ensuring the accuracy and reliability of statistical models.
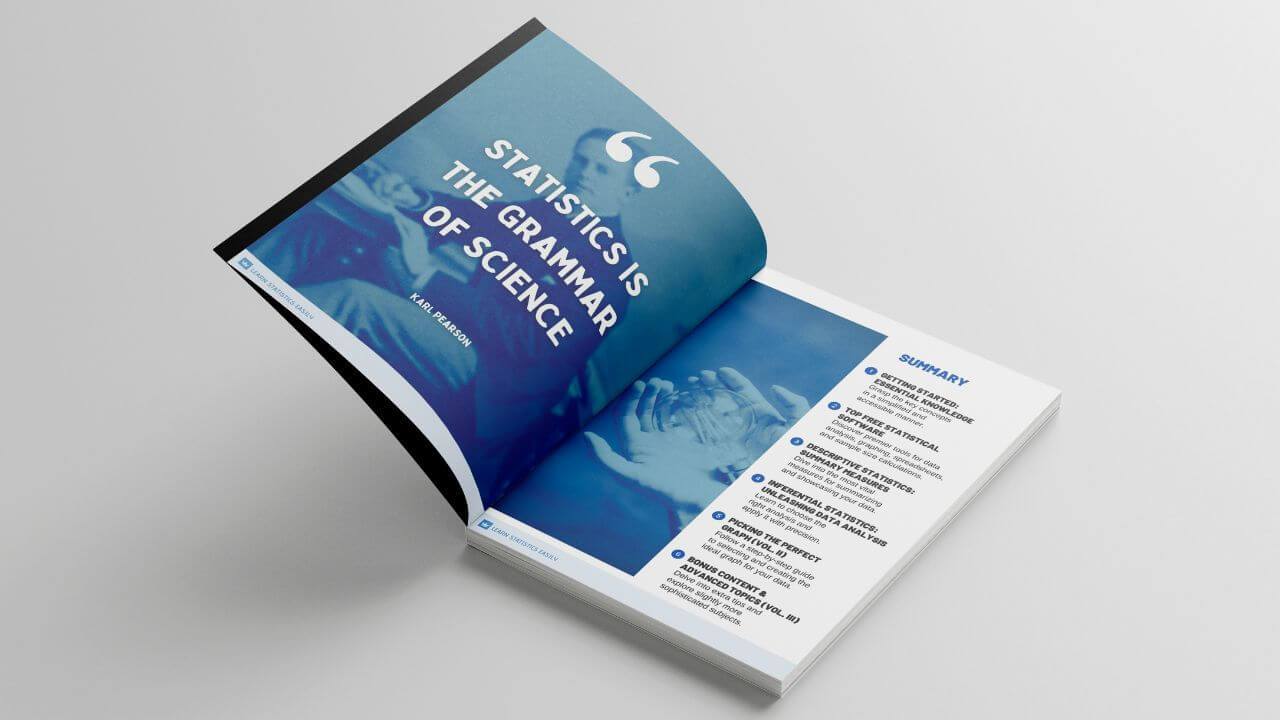
Be a Master of Data Analysis!
Master data analysis techniques and stand out in your research field. Learn in a simple way!
Importance of Identifying Y-Outliers
Identifying Y-Outliers is essential in data analysis as they can skew the results of regression analyses, leading to misleading interpretations. For instance, if a Y-Outlier exists, it can disproportionately influence the slope of the regression line, resulting in an inaccurate model. By detecting and addressing Y-Outliers, data scientists can improve the robustness of their models and enhance predictive accuracy.
Methods for Detecting Y-Outliers
There are several methods for detecting Y-Outliers in datasets. One common approach is the use of statistical tests, such as the Z-score method, which identifies data points that fall beyond a certain number of standard deviations from the mean. Another method is the Tukey’s fences technique, which utilizes the interquartile range (IQR) to determine outlier thresholds. Visual methods, such as box plots and scatter plots, can also be effective in spotting Y-Outliers.
Impact of Y-Outliers on Regression Analysis
Y-Outliers can significantly impact regression analysis by affecting the estimated coefficients and the overall fit of the model. When Y-Outliers are present, they can lead to inflated R-squared values, giving a false impression of a model’s explanatory power. Additionally, the presence of Y-Outliers can increase the residual variance, making it difficult to assess the model’s predictive capabilities accurately.
Handling Y-Outliers in Data Science
Once Y-Outliers are identified, data scientists must decide how to handle them. Common strategies include removing the outliers from the dataset, transforming the data to reduce the impact of outliers, or using robust statistical techniques that are less sensitive to extreme values. The choice of method depends on the context of the analysis and the potential implications of the outliers on the results.
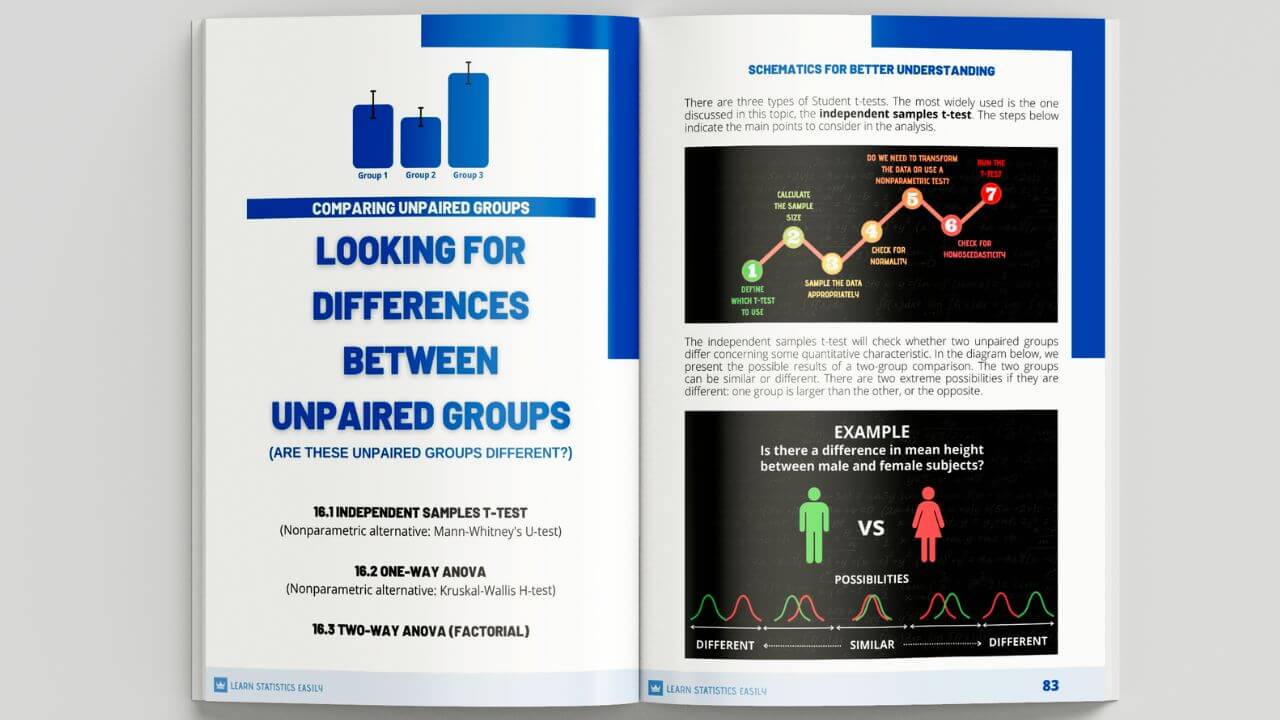
Learn Statistics for Data Analysis!
Demystify statistics and discover how to analyze your data efficiently.
Y-Outliers in Machine Learning
In machine learning, Y-Outliers can pose challenges during model training and evaluation. Algorithms that rely on distance metrics, such as k-nearest neighbors, can be adversely affected by Y-Outliers, leading to poor model performance. Therefore, preprocessing steps to detect and manage Y-Outliers are critical in building effective machine learning models, ensuring that the models generalize well to unseen data.
Examples of Y-Outliers
Examples of Y-Outliers can be found in various fields, such as finance, healthcare, and social sciences. For instance, in a dataset analyzing household incomes, a few extremely high incomes may be considered Y-Outliers. In healthcare, a patient with an unusually high blood pressure reading compared to others in a study could also be classified as a Y-Outlier. Recognizing these examples helps in understanding the implications of Y-Outliers in real-world datasets.
Visualizing Y-Outliers
Visualizing Y-Outliers can provide valuable insights into their distribution and impact on the dataset. Scatter plots, for example, can effectively illustrate the relationship between independent and dependent variables while highlighting any Y-Outliers. Box plots can also be used to visualize the spread of the data and identify outliers based on the IQR. These visual tools are essential for data scientists to communicate findings effectively.
Conclusion on Y-Outliers
In summary, Y-Outliers play a significant role in data analysis and modeling. Their identification and management are crucial for maintaining the integrity of statistical models and ensuring accurate predictions. By employing various detection methods and handling strategies, data scientists can mitigate the adverse effects of Y-Outliers and enhance the quality of their analyses.
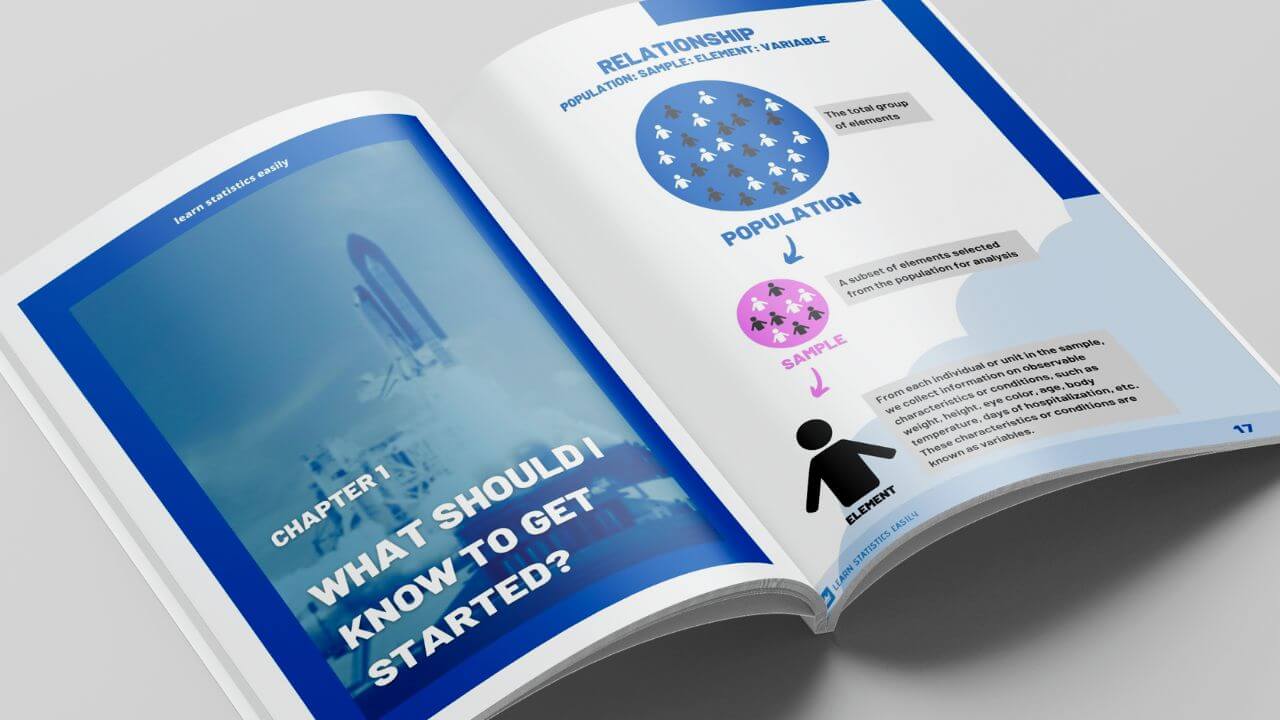
Learn Statistics for Data Analysis!
Demystify statistics and discover how to analyze your data efficiently.