What is: Error Variance
What is Error Variance?
Error variance refers to the portion of the total variance in a dataset that cannot be explained by the model or the independent variables. In statistical analysis, understanding error variance is crucial as it helps in assessing the reliability and validity of the results obtained from a given model. It is essentially the variability in the dependent variable that remains unexplained after accounting for the effects of the independent variables.
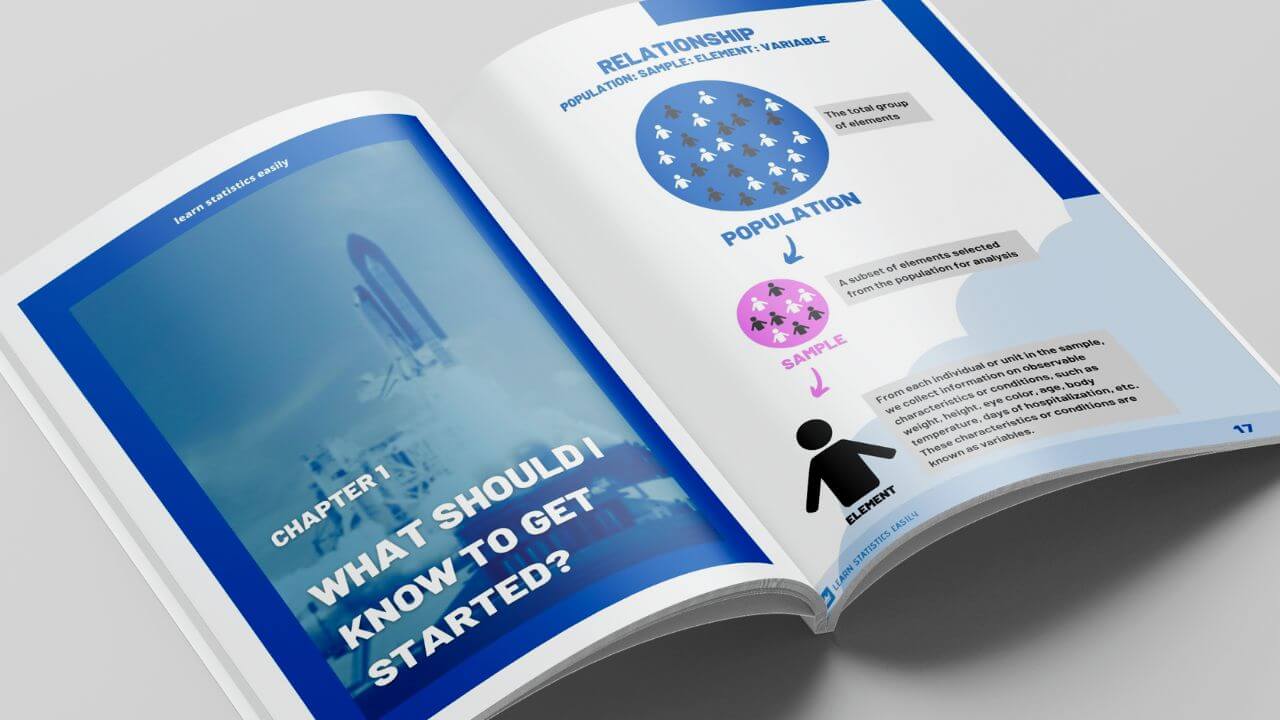
Uncover the Secrets of Data Analysis!
Learn to analyze data like an expert. Transform your scientific work with the power of data analysis!
Understanding Variance in Statistics
Variance is a statistical measurement that describes the spread of data points in a dataset. It quantifies how much the values in a dataset deviate from the mean. In the context of error variance, it specifically focuses on the discrepancies between the observed values and the values predicted by a statistical model. A high error variance indicates that the model does not fit the data well, while a low error variance suggests a better fit.
Components of Total Variance
Total variance in a dataset can be decomposed into two main components: explained variance and error variance. Explained variance is the portion of the total variance that is accounted for by the independent variables in the model. Error variance, on the other hand, represents the variability that remains unexplained. This decomposition is essential for understanding model performance and for making improvements to predictive accuracy.
Importance of Error Variance in Data Analysis
Error variance plays a significant role in data analysis as it impacts the conclusions drawn from statistical tests. High error variance can lead to unreliable estimates of the relationships between variables, making it difficult to identify significant effects. By analyzing error variance, researchers can determine whether their models are adequately capturing the underlying patterns in the data or if further refinement is needed.
Calculating Error Variance
Error variance can be calculated using the residuals from a regression analysis. Residuals are the differences between the observed values and the predicted values generated by the model. The formula for calculating error variance is the sum of the squared residuals divided by the degrees of freedom. This calculation provides a quantitative measure of how much variability in the dependent variable is not explained by the model.
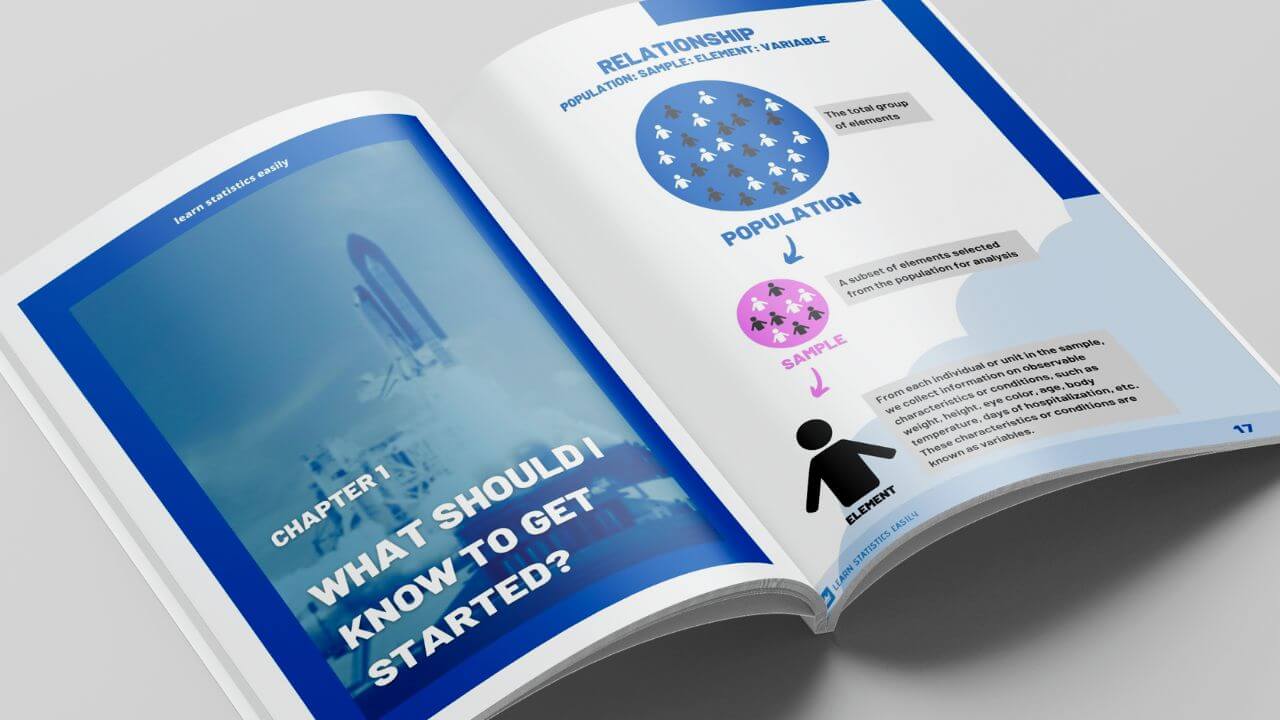
Analyze Data like a Professional!
Unravel the secrets of statistical analysis and enhance your scientific projects. Don’t miss this chance!
Reducing Error Variance
To improve model accuracy and reduce error variance, several strategies can be employed. These include increasing the sample size, selecting more relevant independent variables, and employing more sophisticated modeling techniques. Additionally, data transformation methods, such as normalization or standardization, can help in minimizing error variance by ensuring that the data is appropriately scaled.
Error Variance in Different Statistical Models
Different statistical models exhibit varying levels of error variance. For instance, linear regression models may have higher error variance compared to more complex models like decision trees or neural networks. Understanding how different models handle error variance is essential for selecting the appropriate analytical approach for a given dataset and research question.
Implications of High Error Variance
High error variance can lead to several implications in statistical analysis, including reduced power to detect true effects, increased likelihood of Type I and Type II errors, and challenges in generalizing findings to broader populations. Researchers must be aware of these implications when interpreting their results and consider strategies to mitigate high error variance.
Conclusion on Error Variance
In summary, error variance is a critical concept in statistics and data analysis that reflects the unexplained variability in a dataset. By understanding and addressing error variance, researchers can enhance the reliability of their models and improve the quality of their findings. Effective management of error variance is essential for drawing valid conclusions and making informed decisions based on statistical analyses.
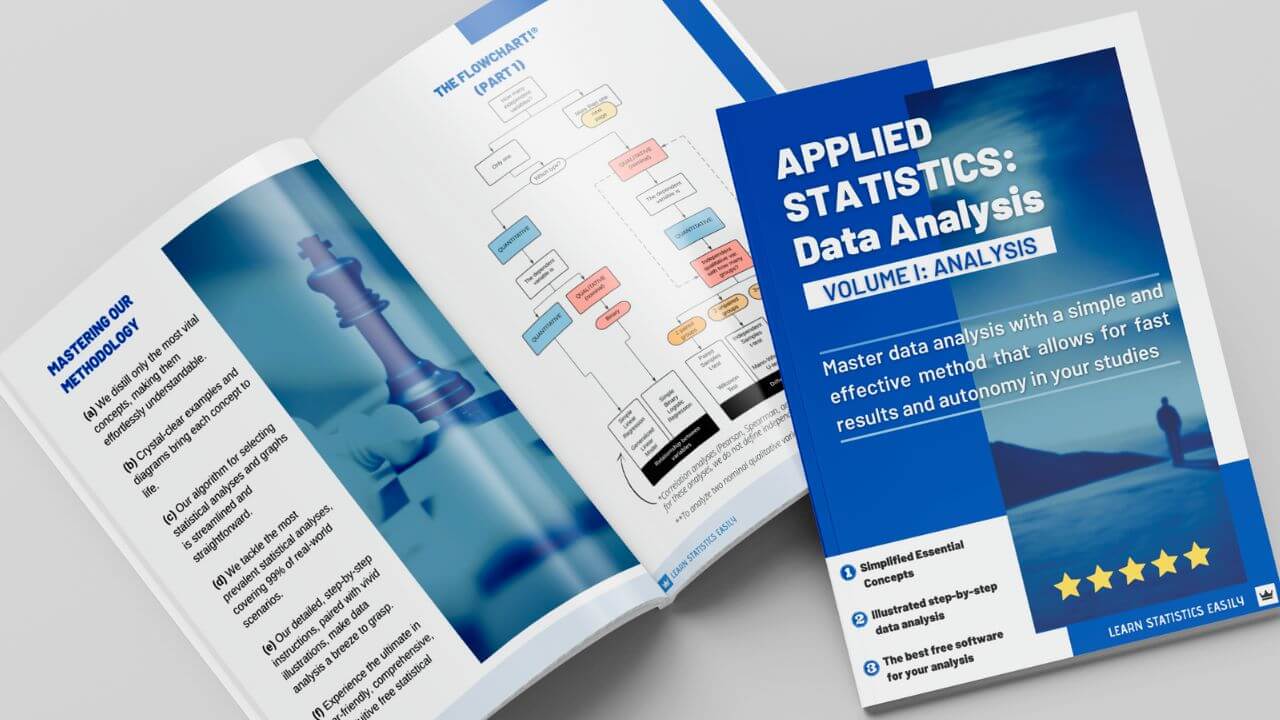
Analyze Data like a Professional!
Unravel the secrets of statistical analysis and enhance your scientific projects. Don’t miss this chance!